Labour MP Challenges Musk's System Gaming
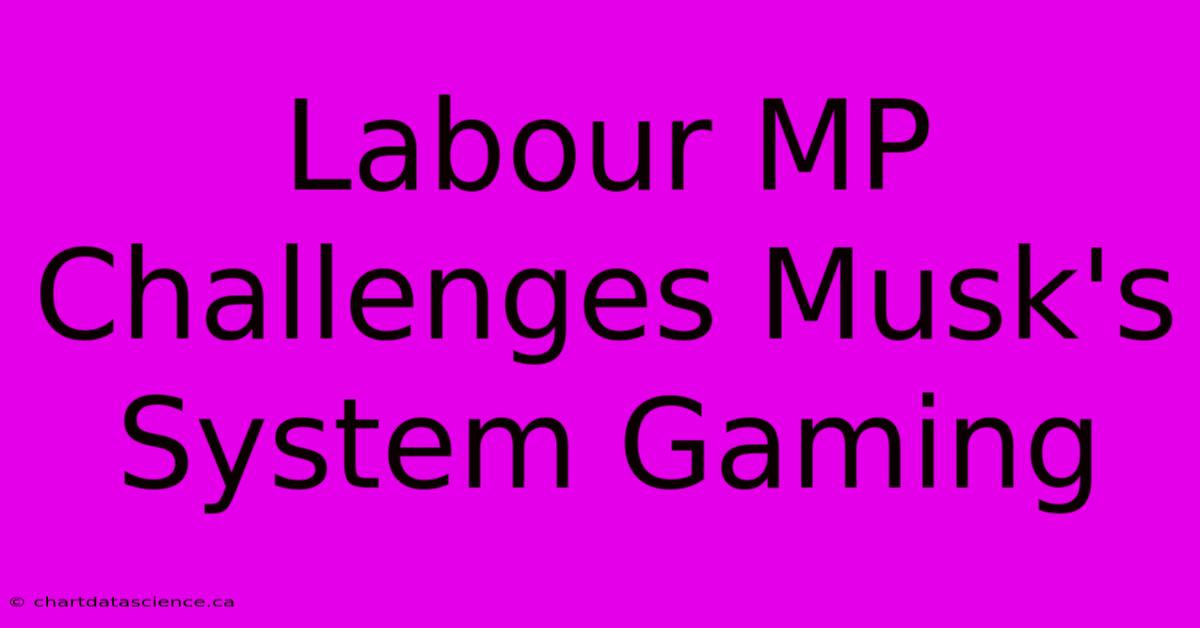
Discover more detailed and exciting information on our website. Click the link below to start your adventure: Visit My Website. Don't miss out!
Table of Contents
Labour MP Challenges Musk's System Gaming: A Deep Dive into Algorithmic Bias and the Future of AI
A Labour MP has recently challenged Elon Musk's companies over concerns about algorithmic bias and the potential for "system gaming," sparking a crucial debate about the ethical implications of artificial intelligence and its impact on society. This article delves into the MP's concerns, explores the complexities of algorithmic bias, and examines the potential consequences of unchecked AI development.
The MP's Concerns: More Than Just a Tweet
The Labour MP's challenge isn't just a fleeting social media post; it represents a growing unease about the power wielded by large tech companies and the potential for their algorithms to perpetuate and amplify existing societal inequalities. The specific concerns raised often revolve around:
-
Algorithmic Bias: The MP highlights the inherent biases embedded within AI systems, arising from the data used to train them. If training data reflects societal biases (e.g., racial, gender, socioeconomic), the resulting algorithms will likely perpetuate and even exacerbate these biases. This can lead to unfair or discriminatory outcomes in areas like loan applications, hiring processes, and even criminal justice.
-
System Gaming: The MP's concerns extend to the potential for individuals or groups to manipulate algorithms for personal gain. This "system gaming" could involve exploiting loopholes or vulnerabilities in the system to achieve an unfair advantage, undermining the intended purpose of the AI. For example, manipulating search engine algorithms for higher rankings or exploiting flaws in social media algorithms to spread misinformation.
-
Lack of Transparency and Accountability: A major concern raised is the lack of transparency surrounding how these algorithms function. The opaque nature of these systems makes it difficult to identify and address bias, making accountability challenging. This lack of transparency also hampers efforts to understand the full impact of these powerful technologies.
Understanding Algorithmic Bias: A Complex Issue
Algorithmic bias isn't simply a matter of faulty programming; it's a reflection of the data used to train the AI. This data often contains inherent biases reflecting societal prejudices. For example:
-
Biased Training Data: If an algorithm used to assess loan applications is trained on data predominantly reflecting the behavior of a specific demographic, it may unfairly discriminate against other demographics.
-
Reinforcement Learning: AI systems can also learn and reinforce existing biases through interactions with users. If a system consistently receives biased input, it will likely perpetuate those biases.
-
Data Representation: The lack of diversity in training data is a major contributor to bias. If the data primarily reflects the experiences of one group, the algorithm will be less accurate and fair in its predictions for other groups.
The Path Forward: Regulation and Ethical Considerations
Addressing the concerns raised by the Labour MP requires a multi-pronged approach:
-
Increased Transparency: Companies developing and deploying AI systems need to be more transparent about their algorithms and the data used to train them. This will allow for greater scrutiny and facilitate the identification of biases.
-
Robust Auditing Mechanisms: Independent audits should be conducted to assess the fairness and accuracy of AI systems, ensuring they don't perpetuate harmful biases.
-
Ethical Guidelines and Regulations: Stronger ethical guidelines and regulations are needed to govern the development and deployment of AI, ensuring responsible innovation and mitigating potential harms.
-
Diversity in AI Development: A more diverse workforce in the AI field is crucial to reduce bias and ensure a wider range of perspectives are considered during development.
Conclusion: The Future of AI and Societal Wellbeing
The Labour MP's challenge serves as a timely reminder of the critical importance of addressing algorithmic bias and preventing "system gaming." The unchecked growth of powerful AI systems without adequate safeguards poses significant risks to societal wellbeing. By fostering greater transparency, implementing robust regulations, and prioritizing ethical considerations, we can harness the potential benefits of AI while mitigating its inherent risks and ensuring a fairer and more equitable future.
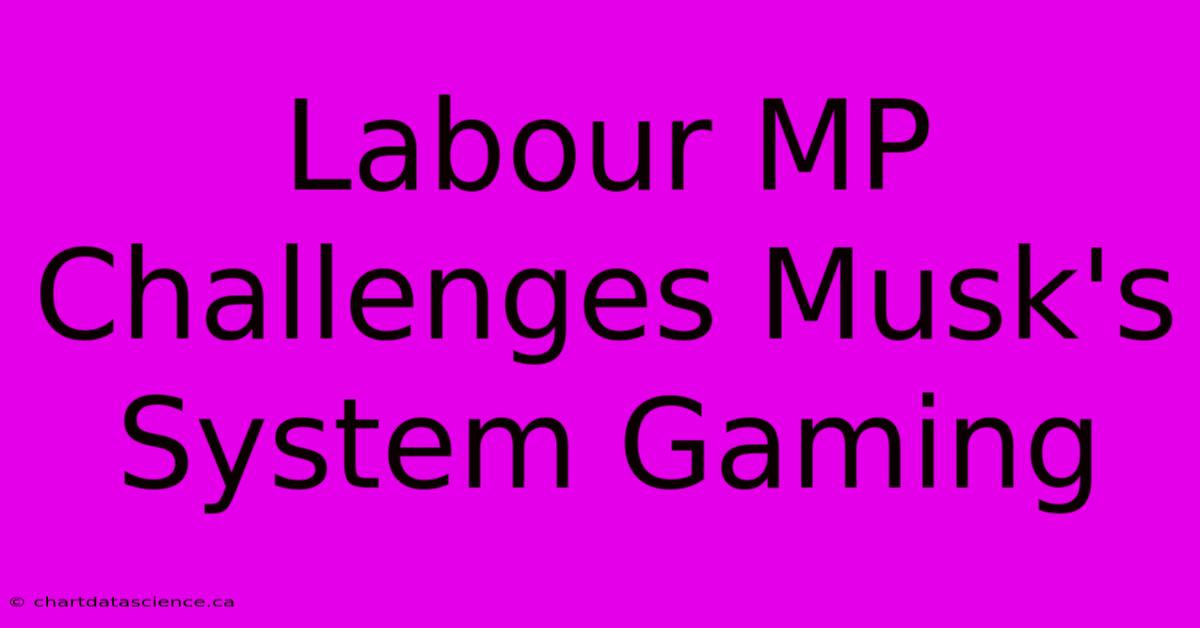
Thank you for visiting our website wich cover about Labour MP Challenges Musk's System Gaming. We hope the information provided has been useful to you. Feel free to contact us if you have any questions or need further assistance. See you next time and dont miss to bookmark.
Also read the following articles
Article Title | Date |
---|---|
Bluey Movie 2027 Release Date | Dec 18, 2024 |
Cyclone Impacts Us Embassy In Vanuatu | Dec 18, 2024 |
Honda And Nissan Explore Merger | Dec 18, 2024 |
Arnolds Surprise Santa Appearance | Dec 18, 2024 |
Musk In Uk Funding Negotiations | Dec 18, 2024 |